Overcoming Diarization Challenges in Dynamic Media
Speaker diarization is the process of partitioning an audio stream containing human speech into homogeneous segments according to the identity of each speaker. It has been a subject of extensive research, primarily focusing on videos within controlled environments such as meeting rooms or phone interviews where the number of speakers typically does not exceed 6.[1] However, with media, it's common to encounter 20-30 speakers in a program. When applying existing models to such videos, they often struggle to identify more than ten speakers.
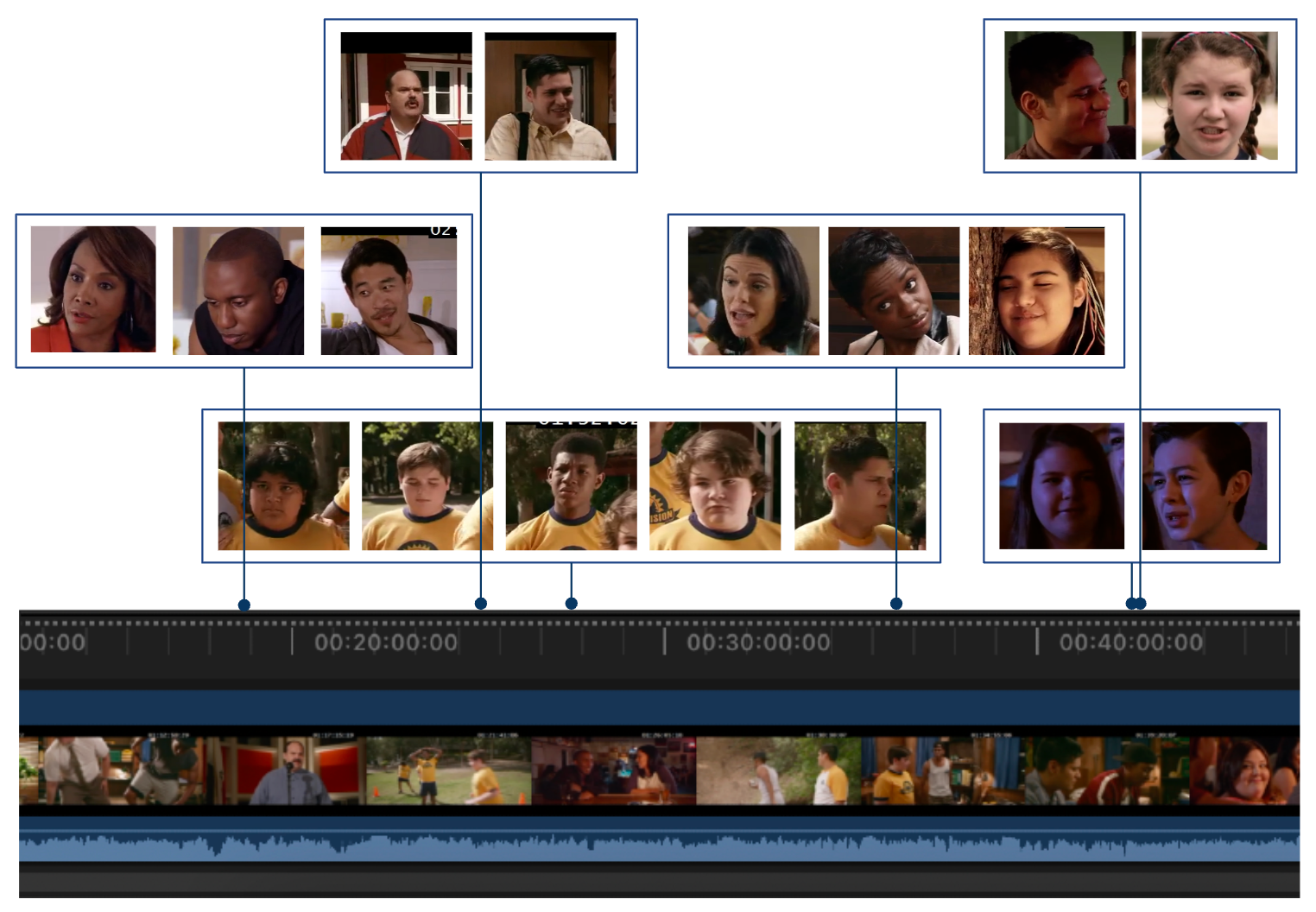
Furthermore, media content often includes scenes such as fights, street scenes, and background music. This complexity makes obtaining clean vocal audio chunks challenging, thus creating a need for a novel approach that can effectively handle these demanding conditions.
Audio-Visual Diarization: A Novel Approach
We pondered the challenge of discriminating between large numbers of individuals solely based on their voices and realized that it could become quite confusing. To address this, we explored the idea of incorporating visual information by simultaneously observing faces while listening to voices. This method, which combines the identification of speakers through both vocal and facial analysis, is commonly known as audio-visual speaker diarization.
This blog introduces our novel approach to audio-visual speaker diarization, showcasing the results of our implementation. We also provide a comparison with the results from other commercial products, discuss the challenges we encountered, and outline the future focus areas for our ongoing work.

In our tests, we obtained a 91.4% accuracy for transcribing the first episode of Friends, surpassing other commercial Speech-to-Text (STT) service providers. Sxxx provided an accuracy of 85.4%, Axxx achieved 80.7%, and Gxxx fell below 70%. Accuracy measurement involves deducing the speaker by analyzing the frequency of results attributing speech to a specific individual, assuming it, and counting instances of contradiction.
Constructing a Character List for Improved Diarization
The core concept revolves around initially identifying all speakers in the media and extracting their facial and vocal characteristics. This creates a character list akin to those found in media, encompassing not only faces but also vocal traits.
Consequently, when a vocal segment is detected in each subtitle, it can be matched to someone in this pre-established character list. Constructing this list in advance allows us to narrow down the set that requires categorization. Without this pre-built list, distinguishing whether a voice is new or belongs to someone encountered before becomes challenging. This is particularly true since current state-of-the-art vocal similarity measures often fall short in delivering accurate results, making the problem more complex.
An additional aid for models to accurately discern individuals involves using facial features as a hint. In cases where vocal similarities are nearly identical for two different persons, comparing the detected face with the faces in the candidate speakers list provides valuable additional information. Given that speakers are more likely to have their faces shown on screen when speaking, this further assists in resolving the identification challenge.
Enhancing Identification with Facial Analysis
To delve into more intricate details regarding the construction of the character list, it's essential to align the speaker's voice with their faces. This is achieved by collecting all faces when a voice is detected. However, it's worth noting that sometimes the face captured may not belong to the person speaking but to someone engaged in the conversation.
Voice-Face Synchronization and Lip Movement Detection
To establish a robust connection between face and voice, we incorporated lip movement detection. This ensures that the detected face corresponds to the voice, as the lips are observed moving during the vocal expression. Subsequently, we clustered all voice-face pairs within a predefined hyperparameter threshold, determined through trial-and-error with 20 media cases where we manually labeled ground truths. Additionally, it’s noteworthy that if a list of characters, accompanied by either faces or voices, is provided by the user, it could serve as valuable clues for the system to create a more accurate character list.

Clustering Voice-Face Pairs and Speaker Labeling
Once the character list is constructed, the next step involves labeling the speaker for each segment. In fortunate scenarios, the face's lips move in sync with the heard voice, allowing us to confidently use the face as a reliable hint. However, if the face captured does not belong to the speaker, there's still a high probability that the actual speaker's face was shown within the last 30 seconds. Therefore, we utilize all faces displayed in this recent time window as a reference for comparison with the character clusters.
Blending Voice Similarity Models for Enhanced Accuracy
Beyond relying solely on facial hints, we have developed a method to enhance the accuracy of computing voice similarity. Recognizing that each voice similarity model has its unique characteristics, we realized that blending them together with an appropriate ratio could yield an improved final result. To determine this optimal ratio, we conducted experiments with 20 media cases that we manually labeled, identifying the most effective blending approach.
Speed vs. Accuracy: Optimizing Video Processing
Implementing this strategy came at the cost of sacrificing speed. The necessity to extract all video frames and detect faces in each frame significantly impacted processing time. We initially explored a method of sampling frames without considering all of them, but this resulted in a noticeable decrease in accuracy. Consequently, we pursued several alternative approaches that maintained accuracy.
Firstly, we limited face detection to areas where faces were identified in earlier frames, thereby reducing computational requirements. Secondly, we resized the image to a 480p resolution, as this was generally sufficient for identifying someone's face in most cases. Additionally, to enhance efficiency, we implemented parallel processing pipelines for independent tasks, such as audio and face processing, allowing them to be executed simultaneously.

Future Directions in Speaker Diarization in Media
We've examined instances where diarization still poses challenges. These are primarily brief exchanges, such as 'oh' or 'hi.' Additionally, complexities arise when the original speaker utters words in varying tones for emphasis or sarcasm. While audio or visual context might assist in these cases, leveraging a large language model could prove beneficial. This model could predict who is likely to utter specific words based on the conversational context.
Furthermore, we observed variations in the quality of voice similarity calculation models across different languages. This discrepancy may arise from variations in the datasets used for training, potentially favoring certain languages over others. Adjusting the blend ratio in accordance with each language might be a plausible solution.

The significance of speaker diarization is expected to grow over time, given that identifying the current speaker serves as the foundation for various media investigations. While some entities concentrate on scenarios within controlled environments, delving into cases within media is valuable due to its proximity to real-world situations. Once this technology is thoroughly developed, its applicability will extend to other domains.